Since the customer experience becomes more crucial, analytical tools and algorithm machines to optimize this become more advanced yet popular nowadays. They evolved as an independent research field in the 90s. Such systems, arguably, thanks to their ability to analyze a customer’s purchase history, can play an important role in increasing sales, introducing new products, and channelizing purchases in order to manage the supply chain.
These state-of-the-art search engines are used in many situations, and the simplest ones we are all familiar with are song and video suggestion systems based on the remnant of the user’s taste in an online streaming website.
What are recommender systems?
Recommender systems are some learning engines, technically look for an individual’s preferences based on his/her previous choices, and through this way are able to support customers in online purchases. More explicitly, a recommender system is a subcategory of a search extraction engine that helps users find the ranking or tendencies of a specific product.
In a plain language, recommender systems are some software agents that can learn the interests, needs, and other momentous individual characteristics to then make suggestions accordingly.
In other word, the recommender systems, designed as programs that are able to introduce the most appropriate items (products or services) to the most eager users (individuals or businesses); monitoring a user from all sides, and based on a multifaceted engagement, even with preoccupation with the people with whom he/she has a close relationship, these systems can discern the individual preferences.
Measuring the interest in an item based on related information about items, users and interaction between items and users is the main application of these smart machines. In fact, they not only improve the user relationship system with a product or service, but because they are competitive oriented, they can push organizations to outmaneuver further than their peers.
There are four types of recommendation process these engines use in order to find out what we want exactly:
- Collaborative
- Content-based
- Knowledge-based
- Hybrid
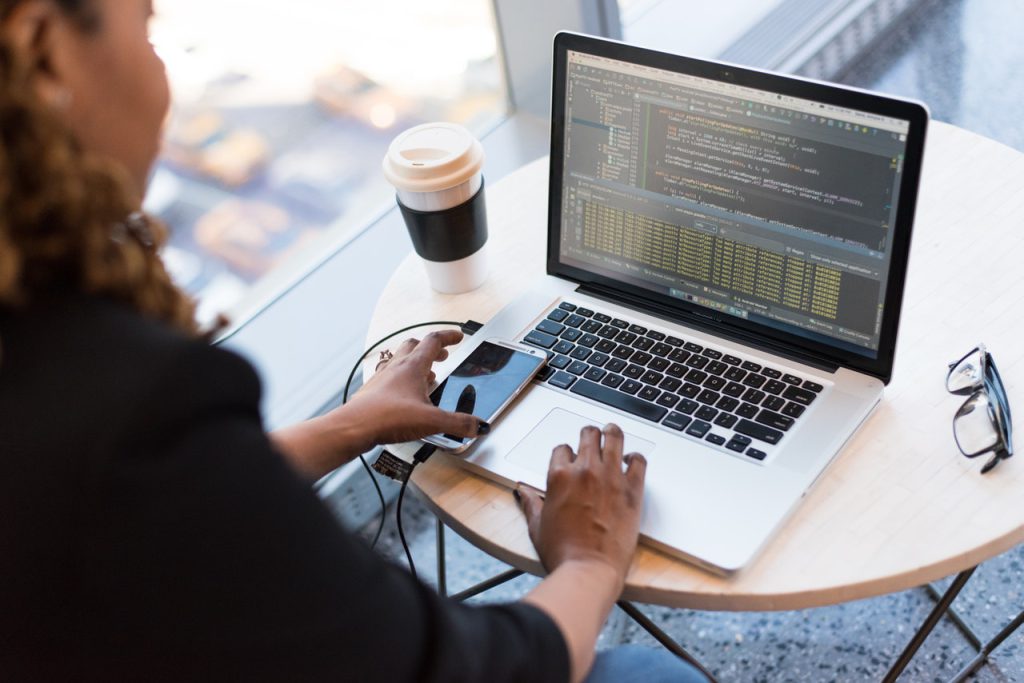
What does a recommender system do?
The recommender system provides offers to a user on a specific item or service, based on a ranked list, which that user is enthusiastic to use. The large amount of data available on a user’s traces allows the system to make predictions about his or her future choices by following in his or her footsteps.
Having used the collaborative filtering, recommender systems underpin for predictive suggestion; they search for emotionally engaging and culturally relevant offers a customer might be satisfied of being acquainted with.
For example, this smart machine memorizes how movies are rated and suggested based on users’ ratings of movie genres to then offer new options and upcoming movies accordingly.
They are also a very efficient tool for managing the information collected through collaboration with partner networks. As a matter of fact, these types of systems glibly facilitate design of surveys, yet providing very limited analytics and insight into customer feedback. In short, it is mostly confined to simple and straightforward trend analysis.
After all, knowing basic concepts in machine learning, statistics, and python are somehow necessary for one who is interested in working and garnering a practical uptake for using or developing recommender systems.
What are the advantages of recommender systems?
Recommender systems are reflective of the aspiring and highly developed domains in today’s information and communication science realm. In this regard, transparency, trust, effectiveness, and satisfaction are characteristics of what called advantages of recommender systems.
The recommender system is considered as a machine learning application that recommends the most relevant products and services to the user. So, it leads customers towards a rigorously engaged state of the mind.
Another advantage of these machines is that they do not need to understand a product and can attract passive customers with previous customer feedback. For example, a recommender system does not know what the Lord of the Rings is talking about, but it does know that it should not recommend the film to anyone who watches political documentaries.
Moreover, these machines accelerate the speed and volume of orders. The faster a customer finds the items they need, the more they will buy. Finally, the most important advantage of these machines is faster communication between companies and suppliers of goods; this can be both C-to-B and B-to-B.
How Recommender Systems Provide Users with Suggestions?
By monitoring the watching and searching habits of similar users the recommender systems make up a habitués and following it they suggest new potential choices. In general, these systems exploit the three themes: users, items, and behaviors; to decide what products to offer to which users. Such smart engines search out to find out what and to what extent the preference an individual may ascribe to an item or a package of service. In fact, every listing you provide for the best sellers is the traditional form of this technology.
Some examples of companies taking the advantage of recommender system
Amazon is the largest user of these systems. Amazon.com also runs a recommendation system in order to identify the online store for every single individual who is somehow engaged.
Another entertainment giant, Netflix, categorizes, deletes or promotes various content based on the user behavior. The company quickly eliminates poor quality content with smart tools that can measure the initial points needed to stay competitive.
There are other fields that need these smart machines a lot and tourism is one of them. Choice Hotels and Gaylord Hotels both use such systems to gather information and emotional analysis of their customers to restore maximum customer satisfaction at the lowest possible cost.
The recommendation system, at the same time, allows customers to be the best service quality inspectors who deal with the service and quality of the rooms, how the staff members treat them, and to some extent the various foods and beverages that make them happy.
Agriculture is also one of the newest businesses that uses this modern method. A recommendation system based on a machine learning approach may be developed that can indicate that the type of vegetable seeds and fertilizer may be used to increase their productivity and thus their income. Many farming companies still use this method, including the famous Chigita banana.
Overall, and to make a long story short, banking, mobile service providers, insurance companies, financial service companies etc., are some examples of professional activities that use the recommender systems nowadays.
Final Words
The main idea of the recommender systems, which is a subclass of information filtering systems, is to advise a customer on how to make their next purchases based on how they rate their products online, which have hitherto been consumed. Such systems not only generate revenue for organizations and vendors, they can also result in interactions between users and others; surprisingly because computers see you, hear you and learn you.